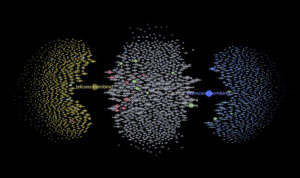
This article from MIT discusses a new paper from University of Waterloo (Ontario), in which researchers suggest that there may be a way to trim down on the number of examples needed to jump-start an AI model. Typically, AI models have to be fed thousands of images to get it to recognize any particular thing. Not only is this very different from how humans learn to identify objects, but also it takes a lot of computation to accomplish. However, in this study, researchers suggested that “soft label” images could be more efficient in enabling AI object-identification. “Soft labels” are ones with a bit of ambiguity–such as a unicorn sharing properties of a horse and a rhino. While the researchers did not build a model, they ran the hypothesis with machine-learning algorithms with promising results.
Read more here: A radical new technique lets AI learn with practically no data | MIT Technology Review